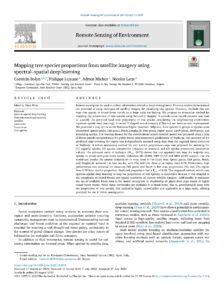
Abstract
Remote sensing can be used to collect information related to forest management. Previous studies demonstratedthe potential of using multispectral satellite imagery for classifying tree species. However, methods that canmap tree species in mixed forest stands on a large scale are lacking. We propose an innovative method formapping the proportions of tree species using Sentinel-2 imagery. A convolutional neural network was usedto quantify the per-pixel basal area proportions of tree species considering the neighbouring environment(spectral–spatial deep learning). A nested U-shaped neural network (UNet++) architecture was implemented.We produced a map of the entire Wallonia Region (southern Belgium). Nine species or groups of species wereconsidered: Spruce genus, Oak genus, Beech, Douglas fir, Pine genus, Poplar genus, Larch genus, Birch genus, andremaining species. The training dataset for the convolutional neural network model was prepared using a mapof forest parcels extracted from the public forest administration’s geodatabase of Wallonia. The accuracy of thepredicted map covering the region was independently assessed using data from the regional forest inventoryof Wallonia. A robust assessment method for tree species proportions maps was proposed for assessing the(1) majority species, (2) species composition (presence or absence), and (3) species proportions (proportionvalues). The achieved value of indicator OA𝑚𝑎𝑗 (0.73) shows that our approach can map the majority treespecies in mixed and pure forest stands. Indicators MS (0.89), MPS (0.72) and MUS (0.83) support that themodel can predict the species composition in most cases in the study area. Spruce genus, Oak genus, Beech,and Douglas fir achieved the best results, with PAs and UAs close to or higher than 0.70. Particularly, highperformance was achieved for detecting Oak genus and Beech in low area proportions: PAs and UAs higherthan 0.70 from the 0.4 proportion. Predicted proportions had a R2𝑎𝑑𝑗of 0.50. The proposed method, which usesspectral–spatial deep learning to map the proportions of tree species, is innovative because it was adapted tothe complexity of mixed forests and spatial resolution of current satellite imagery. Additionally, it optimisesthe use of available forest data in the model conception by considering all pixels from pure stands to highlymixed forest stands. When forest inventories are available in a broad sense, that is, georeferenced areas withthe proportions of tree species, this method is highly reproducible and applicable at a large scale, offeringpotential for use in forest management.
Consultez la notice complète de l’article sur ORBi